1,001 terms for improving AI won’t get us anywhere
An overview of the competing definitions for addressing AI’s challenges

There are a lot of people out there working to make artificial intelligence and machine learning suck less. In fact, earlier this year I joined a startup that wants to help people build a deeper connection with artificial intelligence by giving them a more direct way to control what algorithms can do for them. We're calling it an "Empathetic AI". As we attempt to give meaning to this new term, I've become curious about what other groups are calling their own proposed solutions for algorithms that work for us rather than against us. Here’s an overview of what I found.
Empathetic AI
For us at Waverly, empathy refers to giving users control over their algorithms and helping them connect with their aspirations. I found only one other instance of a company using the same term, but in a different way. In 2019, Pega used the term Empathetic AI to sell its Customer Empathy Advisor™ solution, which helps businesses gather customer input before providing a sales offer. This is in contrast to the conventional approach of e-commerce sites that make recommendations based on a user's behaviour.
Though both Waverly and Pega view empathy as listening to people rather than proactively recommending results based on large datasets, the key difference in their approaches is who interacts with the AI. At Waverly, we’re creating tools meant to be used by users directly, whereas Pega provides tools for businesses to create and adjust recommendations for users.
N.B. Empathetic AI shouldn’t be confused with Artificial Empathy (AE), which is a technology designed to detect and respond to human emotions, most commonly used in systems like robots and virtual assistants. There aren't many practical examples of this today, but some notable attempts are robot pets that have a limited simulated emotional range like Pleo, Aibo, and Cozmo. In software, there are attempts being made to deduce human emotions based on signals like your typing behaviour or tone of voice.
Responsible AI
This is the most commonly used term by large organizations that are heavily invested in improving AI technology. Accenture, Microsoft, Google, and PwC all have some kind of framework or principles for what they define as Responsible AI. (It's interesting that Google’s explanation for the term comes in third on their own search engine.)
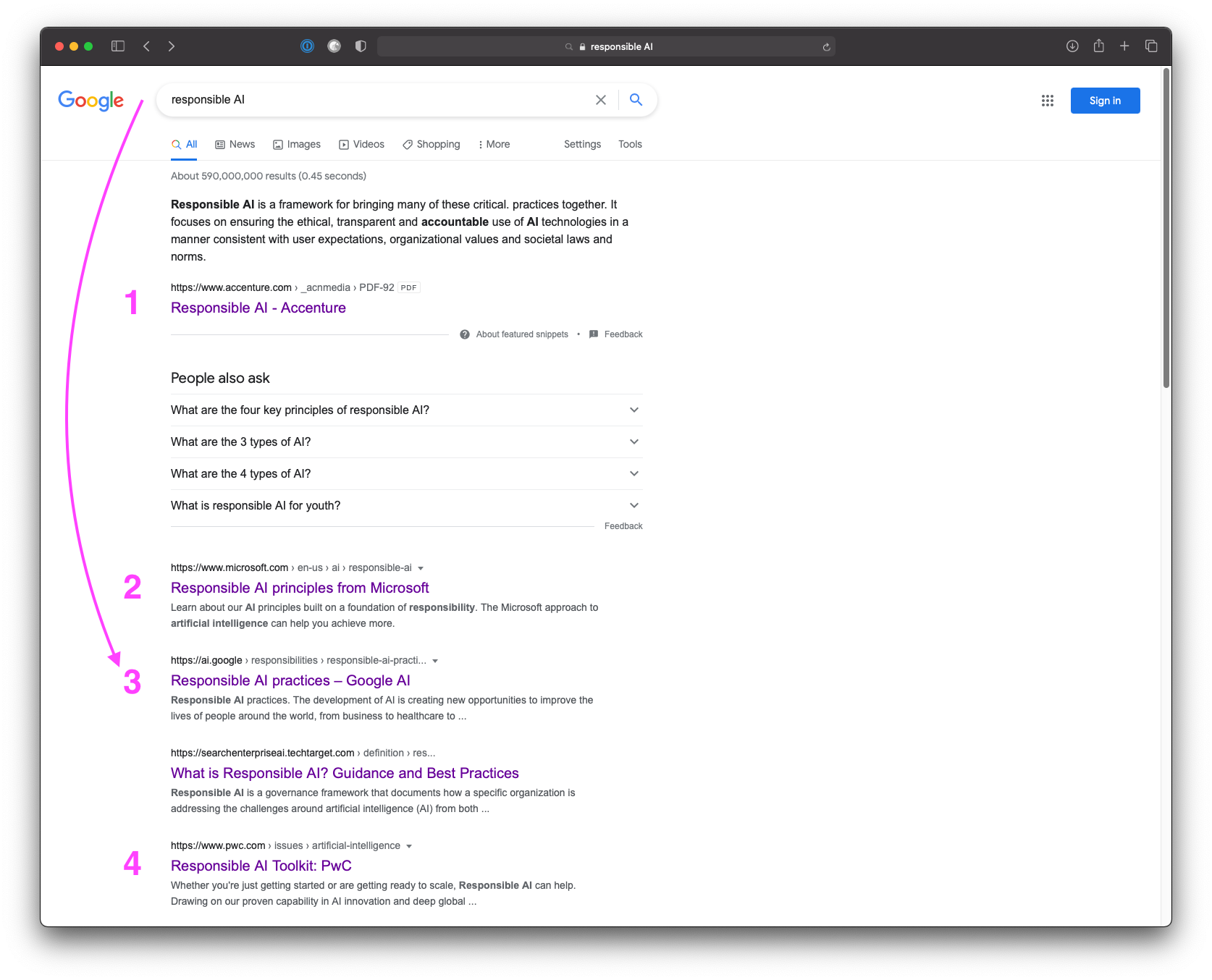
Here's an overview of how each of these companies interprets the concept of Responsible AI:
- Accenture: A framework for building trust in AI solutions. This is intended to help guard against the use of biased data and algorithms, ensure that automated decisions are justified and explainable, and help maintain user trust and individual privacy.
- Microsoft: Ethical principles that put people first, including fairness, reliability & safety, privacy & security, inclusiveness, transparency, and accountability.
- Google: An ethical charter that guides the development and use of artificial intelligence in research and products under the principles of fairness, interpretability, privacy, and security.
- PwC: A tool kit that addresses five dimensions of responsibility (governance, interpretability & explainability, bias & fairness, robustness & security, ethics & regulation).
Though it’s hard to extract a concise definition from each company, combining the different terms they use to talk about "responsibility" in AI gives us some insight into what these companies care about—or at least what they consider sellable to their clients.
AI Fairness
You might have noticed that fairness comes up repeatedly as a subset of Responsible AI, but IBM has the biggest resource dedicated solely to this concept with their AI Fairness 360 open source toolkit. The definition of fairness generally refers to avoiding unwanted bias in systems and datasets.
Given the increasing public attention toward systemic problems related to bias and inclusivity, it's no surprise that fairness is one of the most relevant concepts for creating better AI. Despite the seemingly widespread understanding of the term, there are still much needed conversations happening around the impacts of fairness. A recent article on HBR tried to make the case that fairness is not only ethical; it would also make companies more profitable and productive.
To get a better sense of how the tiniest decision about an AI’s programming can cause massive ripples in society, check out Parable of Polygons, a brilliant interactive demo by Nicky Case.
Trustworthy AI
In 2018, The EU put together a high-level expert group on AI to provide advice on its AI strategy through four deliverables. In April 2019, the EU published the first deliverable, a set of ethics guidelines for Trustworthy AI, which claims that this technology should be:
- Lawful - respecting all applicable laws and regulations
- Ethical - respecting ethical principles and values
- Robust - both from a technical perspective while taking into account its social environment
The guidelines are further broken down into 7 key requirements, covering topics like agency, transparency, and privacy, among others.
Almost exactly a year later, Deloitte released a trademarked Trustworthy AI™ Framework. It's disappointing that they don’t even allude to the extensive work done by the EU before claiming ownership over the term. And then they repurposed it to create their own six dimensions that look a lot like what everyone else is calling Responsible AI. To them, Trustworthy AI™ is fair and impartial, transparent and explainable, responsible and accountable, robust and reliable, respectful of privacy, safe and secure. The framework even comes complete with a chart that can be easily added to any executive's PowerPoint presentation.
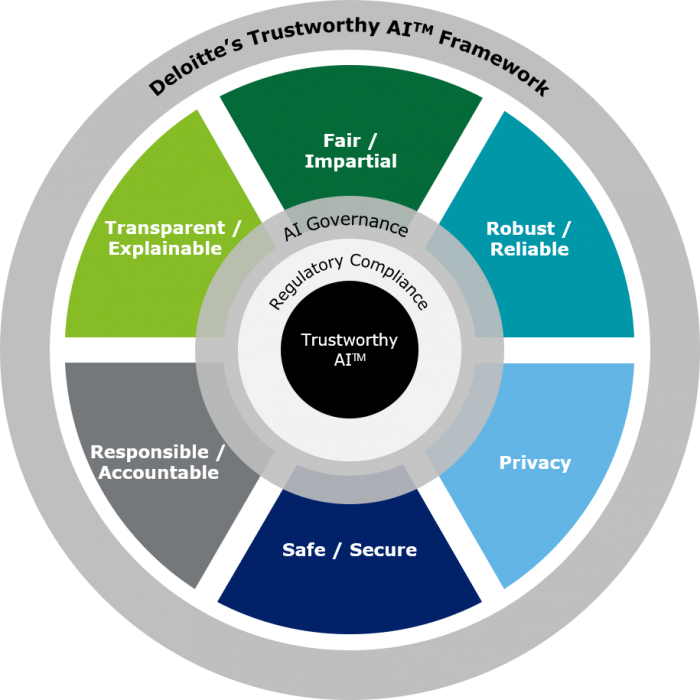
Finally, in late 2020, Mozilla released their whitepaper on Trustworthy AI with their own definition.
Mozilla defines Trustworthy AI as AI that is demonstrably worthy of trust, tech that considers accountability, agency, and individual and collective well-being.
Though they did acknowledge that it’s an extension of the EU's work on trustworthiness, the deviation from the EU-established understanding of Trustworthy AI perpetuates the trend of companies not aligning on communication.
Explainable AI (XAI) and Interpretable AI
All of these different frameworks and principles won't mean anything if the technology is ultimately hidden in a black box and impossible to understand. This is why many of the frameworks discussed above refer to explainable and interpretable AI.
These terms refer to how much an algorithm’s code can be understood and what tools can be used to understand it. They’re often used interchangeably, like on this Wikipedia page where interpretability is listed as a subset of explainability. Others have a different perspective, like the author of this article, who discusses the differences between the two and posits the terms on a spectrum.
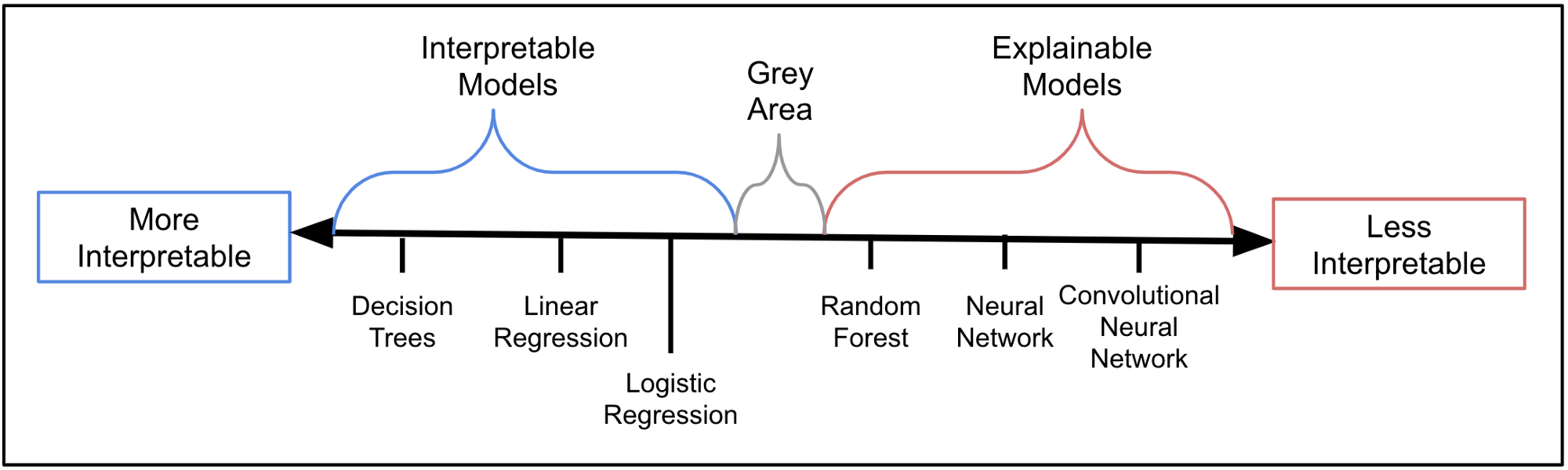
Due to the technical nature of these terms, my understanding of their differences is limited. However, it seems there’s a distinction needed between the term “Explainable AI” (XAI) and “explainable model”. The chart above depicts the different models that algorithms can be based on, whereas the Wikipedia page talks about the broader concept of XAI. At this point, it feels like splitting hairs rather than providing clarification for most people, so I'll leave this debate to the experts.
Competing definitions will cost us
As I take stock of all these terms, I find myself more confused than reassured. The industry is using words that carry quite a bit of heft in everyday language, but redefining them in relatively arbitrary ways in the context of AI. Though there are some concerted efforts to create shared understanding, most notably around the EU guidelines, the scope and focus of each company’s definitions are different enough that it’s likely to cause problems in communication and public understanding.
As a society, we seem to agree that we need AI systems that work in humanity's best interest, yet we’ve still found a way to make it a race to see who gets credit for the idea rather than the solution. In fact, an analysis by OpenAI—the AI research and deployment company whose mission it is to ensure that AI benefits all of humanity—shows that competitive pressure could actually push companies to under-invest in safety and cause a collective action problem.
Though alignment would be ideal, diversity at this early stage is a natural step toward collective understanding. What’s imperative is that we don’t get caught up trying to find terms that make our companies sound good and actually take the necessary steps to create AI systems that provide favourable outcomes for all of us.